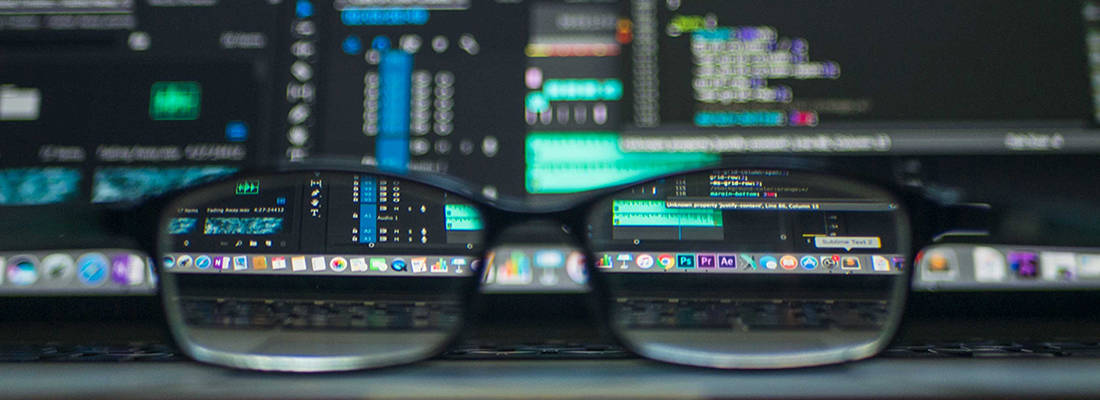
Proof-of-concept to determine feasibility of migrating operations research engine from Maple to Python
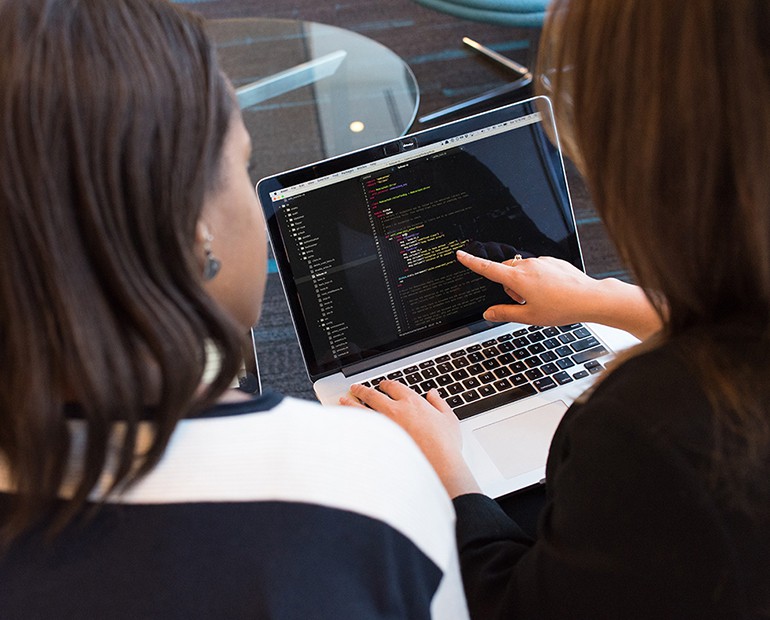
Practitioner-led supply chain consultancy service
Founded in 2013, PerformanSC is a highly successful practitioner-led supply chain consultancy service. PerformanSC works with clients across multiple sectors including healthcare, high tech, retail, food and drink and logistics.
Open-source technology infrastructure
Several of PerformanSC’s data driven performance improvement inputs result from optimisation routines developed on their proprietary operations research toolsets using the Maple programming language. Whilst this technology has served its purpose up to this point, future business needs are likely to require capabilities that solve larger problems with larger data sets.
Phyton is a leading technology within the Data Science area, with most of the recent machine learning and AI work being conducted in and around Python. Working with the software design team in the Design+ Technology Gateway, the company wished to explore the possibility of moving to an open-source technology infrastructure (based around Python programming language), to address two specific supply chain optimisation problems – location-type and transport-type problems.
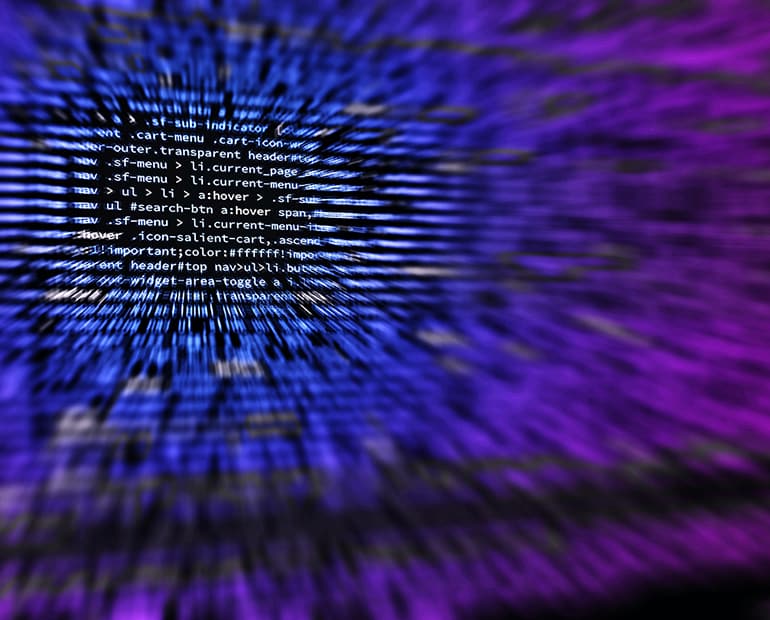
1
Irish company
7
years in business
1
project roadmap
“The team from Design+ provided practical insights into the technology options available to solve the complex data problems that frequently emerge in supply chains. The project provided some valuable insights into how we could deploy our domain expertise and develop our future analytical capabilities.”
Lorcan Sheehan
CEO, PerformanSC
The PerformanSC & DESIGN+ Technology Gateway partnership
When solving location-type problems, the software design team at Design+ successfully demonstrated the feasibility of performing the calculations currently performed by Maple, with technologies based around the Python programming language.
Exploration of Python’s PuLP technology for the transport-type also made progress but presented certain challenges. Trials of Google’s OR-Tools proved more successful with the results closely matching the output produced by the provided worked example (based on the equivalent Maple calculations).
The project highlighted that both operational research problems are solvable with Python (and the concept has been proven), and provided a roadmap to further work required to transform the current proof-of-concept into the next generation, client-facing offering.